Dark matter makes up 27% of the Universe, but it cannot be observed directly due to the fact that it doesn’t emit light. At the same time, its gravitational interaction with the environment makes it possible to detect its effects on a large scale, such as in the halo around galaxies and Einstein rings.
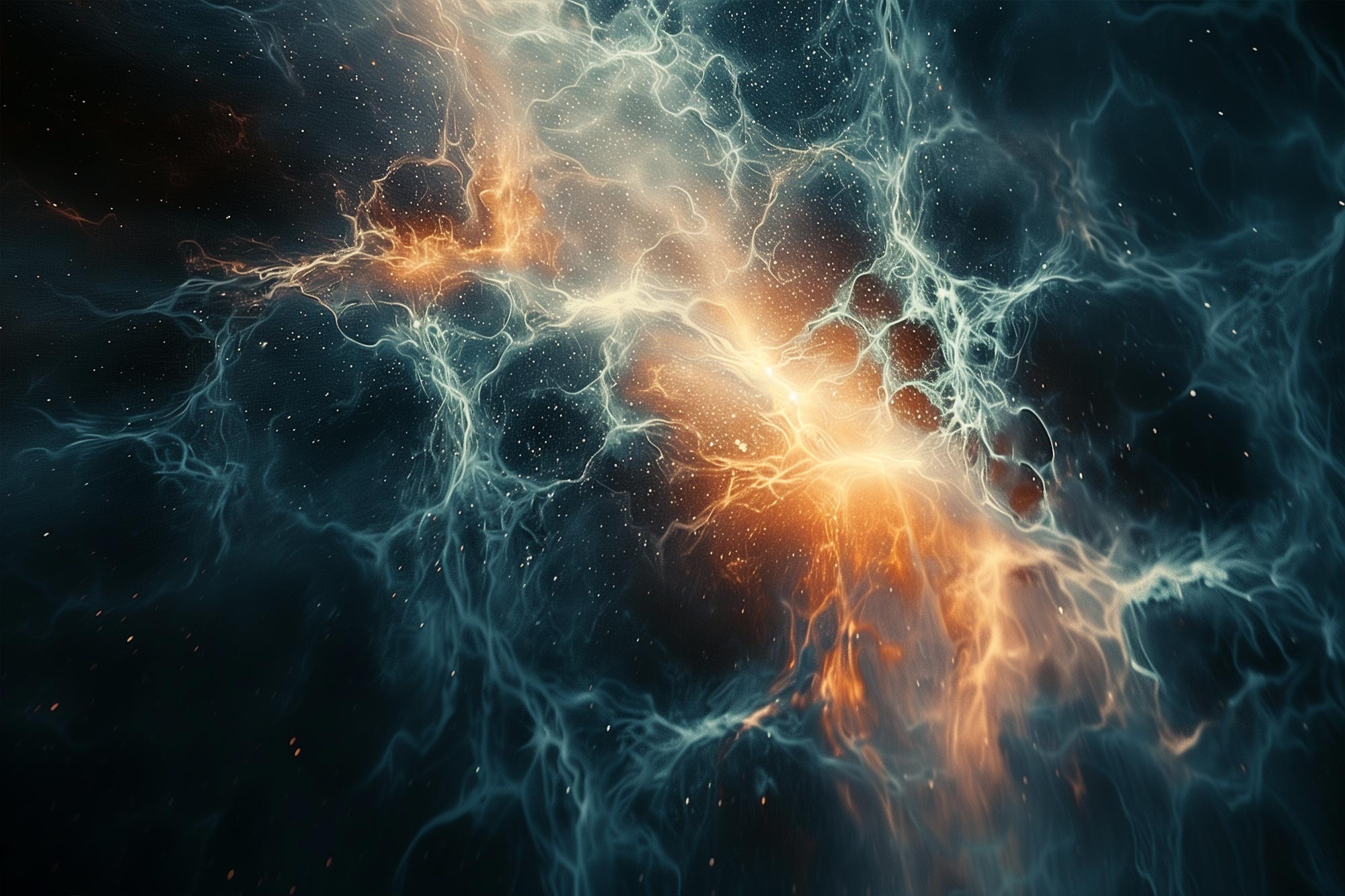
Separating dark matter interactions between each other and other cosmic signals is a difficult task. However, a deep machine learning algorithm using neural networks helps solve this problem by distinguishing the gravitational effects of dark matter from signals from active galactic nuclei with supermassive black holes. A study describing this algorithm is published in the journal Nature Astronomy.
Astronomer David Harvey of École Polytechnique Fédérale de Lausanne has trained a neural network to analyze images from the BAHAMAS-SIDM project, which models the interactions of dark matter with galactic clusters. The neural network, after obtaining the image, learned to separate the dark matter signals from the noise caused by galactic nuclei. Harvey explains that weak lensing differentiates dark matter interactions, while X-ray data helps distinguish astrophysical feedback models.
The most accurate neural network called Inception achieved 80% accuracy in filtering out extraneous noise. This approach could be used in future studies using telescopes like the Euclid Space Telescope, which observes billions of galaxies.
Although scientists still don’t know which elementary particles are responsible for dark matter, artificial intelligence techniques are helping to speed up this important discovery. Thanks to powerful telescopes and neural network-based algorithms, researchers can more efficiently analyze vast amounts of data and move closer to understanding the nature of dark matter.
According to Gizmodo